These days, it is pretty impossible to go through the internet and not come up to term like AI. It’s one of those terms that make every man looking at least 10% smarter when mentioning it, no matter if you don’t know much about it or you are conspiracy theories fan or it’s just interesting to you, repeat AI word whenever you can and you will look so sexy and smart.
So, I decided to write a series about this interesting topic, on the way that will be, I hope, understandable to the major audience. I just want to bust some myths, introduce you and prepare you for the future and maybe to spark your interests a little bit to search about this on the right places, on your own.
What an average person knows about AI:
Ummm man, I watched Terminator and... oh wait, wait, I also watched Matrix, man it’s so good movie. Hey man are you that Nikola guy, which was writing about that Ex Machina movie recently? Oh man, I can’t believe it’s you, I also watched that movie, thanks bro! This AI is dangerous man, we are all going to finish as slaves, that’s some weird stuff man...
Actually, I have to disappoint you (or maybe to encourage you) and say that it is unlikely scenario in our near future. There are a lot of challenges in the field of AI, which have to be completed to consider a machine being on the same level or superior than a man. So relax, we will not finish as slaves...yet.
What a little bit more informed average person knows about AI:
Omg, have you seen that Facebook had to shut down their AI? And... and Google is also developing their AI! Lord knows what they do with my data... I know – I’ll delete my Facebook account! Oh... oh, I’m gonna delete my Steemit account too – man, you’ll never know!
This is justified opinion, because we really don’t know what they are doing with our data and there are big chances that there is some kind of abuse behind everything, but you should know that also those stories are a little bit magnified by the news in order to make sensation. However, the truth is somewhere in the middle.
So before we dive into the story, when and how it all begun, let’s talk about where we can actually use AI today. Potential is limitless and there are numerous examples, but I’ll name just a few:
Autonomous cars – A prototype of this idea is revealed back in 1980s by US DAPRA and it could avoid obstacles and drive off-road in day and nighttime conditions. In 2005, a robot car Volkswagen Touareg, called 'Stanley', won the race on DAPRA grand Challenge. In 2017 Audi claimed that its latest A8 will be the first production car to reach level 3 of autonomous driving (the driver can safely turn its attention away and a car can handle unpredictable situation). In December 2017 Elon Musk announced that it will need about two years more to launch a fully autonomous Tesla on the market.
Robotics – There are military robots that can be used for search or attack, like the PackBot which was originally used in Iraq and Afghanistan.
Games – Everybody heard about legendary chess game between Garry Kasparov and IBM’s computer Deep Blue. Computer could search to a depth up to twenty moves in advance in some situations and won with a result 3.5 : 2.5. Kasparov said that he noticed some deep intelligence and creativity in the machine’s moves. The match was a big sensation and raised value of IBM’s stocks for about 18 billion dollars.
Speech recognition – Recently we could see a new version of Google assistant that can do phone call and appoint a meeting for you. If you haven’t seen it yet, you could do it on this link, it’s quite awesome.
Behind all this things there isn’t some science fiction, but the complex probabilities, mathematics and technology with a little bit philosophy.
Now, do you know how it all started? Let’s make a timeline!
1940s - The birth of AI
The first work which can be related to AI is published by Warren McCulloh and Walter Pitts, and is called "A logical calculus of the ideas immanent in nervous activity". When he was just 12 years old, Pitts found Bertrand Russell’s Principia Mathematica and read all 2000 pages and found couple mistakes in it. He decided to write a letter to Bertrand Russell and amazingly – he wrote back! Russell was so excited and called young Pitts to come to study at Cambridge. Walter couldn’t go, because he was only 12 at that moment, but 3 years later he heard that Russell is going to visit the University of Chicago, so he run away from home to meet him and never came back. On the other side, McCulloh was 25-years older, philosopher and a poet who was deeply interested in neurophysiology. They met each other in Chicago and started working together on modeling human brain as a logical machine. They come up with an idea about model of artificial neural network, where every neuron has two conditions – on and off, and it can be turned on by a stimulus from nearest neurons. They showed that every calculable function could be calculated using this network and that all logical statements could be modeled by this network!
Beside those two, many others were researching this topic and maybe the most influential work at that time was Alan Turing’s “Computing Machinery and Intelligence”. Turing is considered as a father of computer science (you’ve maybe heard about Turing’s Machine) and in this work he announced his famous Turing Test. The test is quite simple – a man is talking via keyboard and screen with a man and also with a machine and after the conversation if he can’t tell which one was machine, we can tell that machine is intelligent and it passed the test. He also introduced things like machine learning and algorithms.
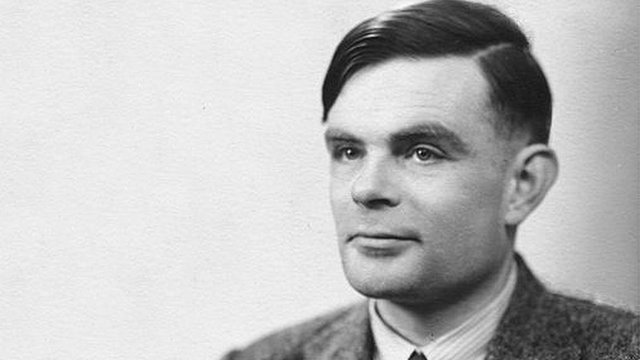
Alan Turing Source
After Turing, Donald Hebb showed one simple rule that helps neural network to get stronger - if one neuron is often stimulating other, then their connection will get stronger, otherwise it will get weaker. This rule is still using to this day and it’s known as Hebb’s learning rule.
1950s - AI officially became a new area of science
At that time, one significant thing happened: It was decided to be organized 2-months workshop in Dartmouth, that will gather American researchers together to discuss and research about AI. Original statement was:
We propose that a 2 month, 10 man study of artificial intelligence be carried out during the summer of 1956 at Dartmouth College in Hanover, New Hampshire. The study is to proceed on the basis of the conjecture that every aspect of learning or any other feature of intelligence can in principle be so precisely described that a machine can be made to simulate it. An attempt will be made to find how to make machines use language, form abstractions and concepts, solve kinds of problems now reserved for humans, and improve themselves. We think that a significant advance can be made in one or more of these problems if a carefully selected group of scientists work on it together for a summer.
Nothing special happened in Dartmouth that year, but all big names connected then and later continued to work together and dominated in this area for about 20 years.
1960s – Big enthusiasm
Somehow, this area started exponentially expanding and we had many scientists, who were working parallel in significant projects in this period. I’ll mention just some of them:
Bernie Widrow and Frank Rosenblatt continued developing Hebb’s work and introduced us with adaline and perceptrons.
In IBM there were developed some of first programs which could do “intelligent things”. For example, geometry Theorem Prover had ability to prove some theorems which many students couldn’t.
John McCarthy founded laboratory for AI on Stanford.
David Huffman worked on microworld of blocks, where robotic arm had to recognize certain object and carry it to the box.
1970 – AI hit its plateau
Scientists were largely encouraged by early accomplishments, so they were giving sensational interviews with almost impossible predictions about future development of artificial intelligence. Following years, unfortunately, denied all those crazy statements, because the first challenges in this area were coming on the surface.
First problem was that machines weren’t actually able to think and conclude new things, they were just manipulating the data in some kind of way. There is one good and funny example during the cold war, when American government tried on all possible ways to translate Russian scientific documents after Sputnik launching. US National Research Council invested large amounts of money to projects which were developing machine translators (something like grandfather of Google Translate) and after years of developing, translator succeeded to translate sentence “the spirit is willing but the flesh is weak” from Russian to English as “the vodka is good but the meat is rotten”. After that fail, US government aborted all investments in future projects about machine language translation. That is the typical example that AI must use and connect a lot of different knowledge in order to work, just like humans.
Another type of problem was that computers were able to work and do some operations in small domains, but in domains with larger number of elements searching through the all possible combinations of elements was terrible. For example, using BFS search with today technology through the tree where every node has ten successors, it will need:
- on depth 2 : about 0.11 milliseconds and 107Kb of memory (110 nodes)
- but on the depth 8 : about 2 minutes and 103 Gb of memory (10^8 nodes)
- and on the depth 14 it will need 3.5 years and 99 petabytes to check all possible combinations (10^14 nodes)
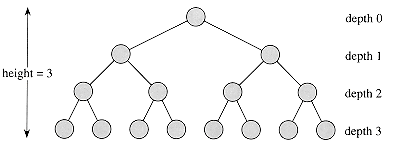
Source
Thanks to this all the hype about AI started to fade away slowly in the middle 70s.
1980s – Commercialization of AI
Next to US, two more countries joined in the artificial intelligence race – England and Japan. US had first successful commercial intelligent system R1 which succeeded in few years to save about 40 million dollars for its company Digital Equipment Corporation. Soon, every major corporation in America had its own group for researching intelligent systems.
A lot of this projects failed, but one group of researchers “discovered again” backpropagation algorithm. The algorithm was originally discovered back in 1969 but somehow wasn’t gain much attention and soon was forgotten.
The idea behind this algorithm is to first set all weights between neuron connections as random and for some input to try to calculate output on your training set. After that, you compare your output with the real values and try to see the error. Once the error is calculated, you go backward layer by layer and use that error as a signal which will fix those weights.
This gave a new hope in researching neural networks and soon AI strongly adopted science methodology and was standardized – every assumption in order to be accepted, had to go through serious experiments and the result had to be analyzed by statistic methods.
1990 – present: Rise of the Internet
Earlier, scientist were trying to make perfect algorithm – depending on the problem, they would always choose which algorithm could give best performance. With the rise of the internet, technique of training AI became a little bit different.
A large amount of data is accessible now, so the training sets can be now multiple times bigger than before. For example, experience showed that extraordinary algorithm with smaller set of data for training can give worse results than average algorithm with big training set!
Now, AI is directed in building intelligent agents – it’s something which can observe its environment through sensors and based on that can conclude something and then to do some action through actuators. A man is some kind of agent – it has eyes and ears as sensors and after some thinking, it can do some action with its arms and legs as actuators.
AI made a really good progress during its short history, but there is no doubt that there are many challenges waiting to be solved and I would love to finish this text with Turing’s quote:
We can see only a short distance ahead, but we can see that much remains to be done!
Sources:
Artificial Intelligence: A Modern Approach, Peter Norvig and Stuart Russell
Wiki Dartmouth workshop
Wiki Autonomous Cars
Wiki Deep Blue
Very interesting read. You have earned a new follower. I look forward to reading the related posts, which I will do now. :)
Oh, I see that you have read older parts, that's nice :)
Thanks, there will be certainly more articles on this topic, stay tuned :)
Vaš post se nalazi u ovonedeljnoj promociji autora - a mi vam od srca želimo da nastavite da stvarate sjajne sadržaje na blogu. Vidimo se uz neki novi teskt ili na našem Discord kanalu :-) Srećno blogovanje!Pozdrav @nikolanikola
Sjajan post, svaka čast :-)
Hvala, bice ih jos :)
Radujem se unapred 👍
Hi nikolanikola,
LEARN MORE: Join Curie on Discord chat and check the pinned notes (pushpin icon, upper right) for Curie Whitepaper, FAQ and most recent guidelines.
Congratulations! this post got an upvote by @steemrepo and was manually picked by the curator @yanosh01 to be added on STEEM REPOSITORY, simply comment "YES" and we upload it on STEEM REPO Website.
Want to know more about the Steem Repo project? Contact us on Discord
Yes
I'm surprised it was only mentioned about machine learning in the 1940's as thats starting to be a buzz word as of recent. I know its wikipedia but still some decent insight https://en.wikipedia.org/wiki/Machine_learning
Machine learning
Machine learning is a subset of artificial intelligence in the field of computer science that often uses statistical techniques to give computers the ability to "learn" (i.e., progressively improve performance on a specific task) with data, without being explicitly programmed.
The name machine learning was coined in 1959 by Arthur Samuel. Evolved from the study of pattern recognition and computational learning theory in artificial intelligence, machine learning explores the study and construction of algorithms that can learn from and make predictions on data – such algorithms overcome following strictly static program instructions by making data-driven predictions or decisions, through building a model from sample inputs. Machine learning is employed in a range of computing tasks where designing and programming explicit algorithms with good performance is difficult or infeasible; example applications include email filtering, detection of network intruders or malicious insiders working towards a data breach, optical character recognition (OCR), learning to rank, and computer vision.
Ovaj post je vrhunski napisan!
Baš sam uživala čitajući i sutra planiram da ga pročitam grupi dece. Kapiram da ću ih oduševiti. Hvala @nikolanikola!
Hvala tebi!
To bi bila velika cast, javi kako su klinci reagovali :)