Artificial Intelligence (AI) has emerged as a transformative force in modern society, driving innovations and advancements across numerous industries. There are two major approaches to AI: Statistical-AI and Logical-AI. Let's elucidate the fundamental differences between these approaches, exploring their strengths and weaknesses.
Statistical-AI:
Statistical-AI, also known as machine learning, is an approach that empowers machines to learn patterns and make predictions from vast amounts of data. This technique does not rely on explicitly programmed rules but instead employs algorithms to identify statistical patterns in data. The cornerstone of Statistical AI is the utilization of training data, enabling AI systems to generalize and make decisions on unseen inputs. It has proven to be remarkably successful in applications such as natural language processing, computer vision, and recommendation systems.
One of the primary strengths of Statistical-AI lies in its adaptability to handle complex and unstructured data. By leveraging deep learning models, such as neural networks, it can extract intricate features from raw data, unlocking previously unimaginable capabilities. Moreover, Statistical-AI's ability to continuously improve through feedback loops makes it highly valuable in dynamic environments.
However, Statistical-AI does have its limitations. Its performance heavily relies on the quantity and quality of training data, and it may struggle in scenarios where data is scarce or biased. Furthermore, the "black-box" nature of some deep learning models raises concerns about interpretability and explainability, hindering its adoption in safety-critical domains.
Some examples of real world applications:-
- Image Recognition: Identifying objects and scenes in images using deep learning models.
- Natural Language Processing: NLP tasks like text classification, machine translation, and sentiment analysis.
- Recommender Systems: Offering personalized recommendations based on user behavior and preferences.
- Autonomous Vehicles: Enabling self-driving cars to navigate and make real-time decisions.
- Sentiment Analysis: Analyzing social media data to gauge public sentiment.
Logical-AI:
Logical-AI, also referred to as symbolic AI, adopts a more rule-based and deductive reasoning approach. It entails encoding human knowledge and expertise into a structured format, represented through symbols, rules, and logical relationships. This enables AI systems to reason, infer, and draw conclusions based on the underlying knowledge base.
One of the key strengths of Logical AI is its transparency and explainability. The explicit representation of rules and the traceability of each decision make it easier for experts and users to comprehend the AI system's behavior. Additionally, Logical-AI can be more reliable in domains where explicit, logical rules govern the outcomes, such as in certain expert systems and theorem proving.
However, Logical-AI faces challenges in dealing with ambiguity and uncertainty, which are inherent in real-world data and human language. Capturing and formalizing all human knowledge into a logical framework can be an arduous and time-consuming task. This approach may also struggle to handle large-scale, complex datasets, limiting its applications in data-rich domains.
It also should be noted that Logical-AI would be able define a Statistical-AI, but not the other way around.
Some examples of real world applications:-
Expert Systems: Logical-AI is extensively used in expert systems, where human expertise is encoded in the form of rules to solve complex problems in specialized domains like medicine, engineering, and finance.
Theorem Proving: In mathematics and formal logic, Logical-AI is employed to prove mathematical theorems using logical inference and deduction.
Knowledge Graphs: Logical-AI techniques are utilized to create and reason over knowledge graphs, representing facts and relationships in a structured manner.
Automated Reasoning: Logical-AI can be applied in systems that automatically reason and draw conclusions based on given facts and rules.
Semantic Web: Logical-AI techniques are employed to make sense of web data and ensure interoperability among different web resources.
Comparison table
Here's a table on their differences.
Statistical-AI | Logical-AI | |
---|---|---|
Approach | Learns from data patterns | Relies on explicit rules and logic |
Knowledge Base | Implicit in large datasets | Explicitly encoded knowledge |
Decision-making | Data-driven and probabilistic | Rule-based and deductive reasoning |
Training Data | Requires extensive labeled data | Relatively small, curated dataset |
Transparency | Often considered a "black box" | Transparent and explainable |
Complexity Handling | Excels with unstructured data | Struggles with ambiguity and uncertainty |
Domain Suitability | Widely used in various domains | Well-suited for rule-based systems |
Strengths | Excellent at pattern recognition | Offers human-interpretable results |
Weaknesses | May lack interpretability | Limited in handling complexity |
I've just finished watching the one hour+ interview by David Shapiro on Youtube. The concept of logical AI sounds really convincing. Also quite shocked to see the number of views for official clips being so low... Need to study much more... Although not too easy to grasp. 😵
Yeah that was a good interview. We really have no idea why the thing we thought is the best in all of crypto is still like the least popular haha xD but there's just too much thinking involved. Better to have the testnet so ppl play around with it
Before the testnet arrives, are you aware of some articles or videos that give some simple examples that can demonstrate how tau works? Like how to transform natural language to controlled language or how some simple pieces of statements in controlled languages can be sorted to conclusion by machine computing etc... I seem to understand the concept but need some concrete cases to help me visualize what a tau future would be like.
check out tml.tau.net, go to the intro folder and check out each one (as per screenshot below)
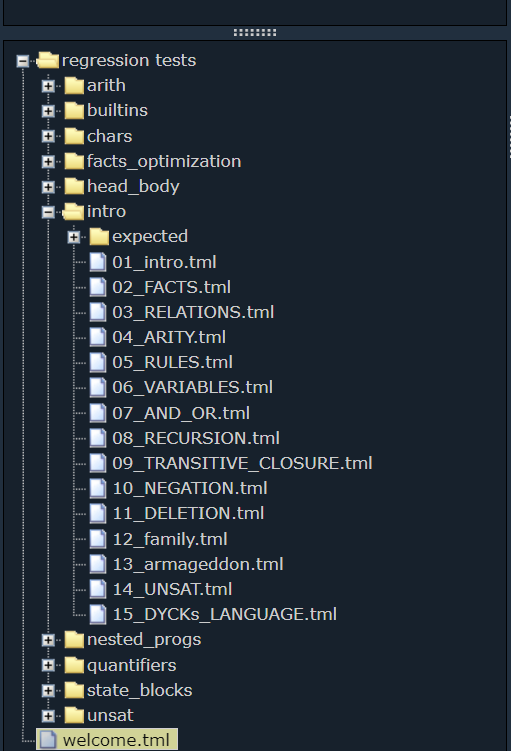
Taken from https://hive.blog/tauchain/@trafalgar/the-power-of-tau-scaling-the-creation-of-knowledge
Can also check out https://www.youtube.com/@LEARNTAU/videos (scroll down)
I can't recall if there's anything that is more visual atm. Maybe you can drop by the telegram and ask? https://t.me/tauchain
Wow, thanks! that's a lot.
For tml website, oh no, mostly are in the form of codes. Not for me at the moment😆.
Re Traf's post six years ago, WOW! I cannot recall that I'd read it but I did vote for it. Six years... it's like eternity in crypto but they are still here at the least. I bet no one who commented in that post could foresee this situation.
It seems that @dana-edwards was the first to talk about Tau on Steem back then? and then you and traf? It's a pity to see him inactive for two years. For Steem/Hive as a blogging chain that deals with 'words' it would be awesome to combine with Tau.
Those videos seem not so helpful. I guess they have not yet in the phase of serious marketing or awareness promotion.
Will try asking on telegram once I have done more research. By the way, I think I should finished reading the white paper first. I just checked, only a small part of it is formula or codes😄.
Thanks for all these 🆃🅰🆄 information. Much appreciated🙏.
I think roelandp is the only top witness following the project as well, not sure. I think it'll be a useful tool for what we have here on Hive, although that remains to be seen. it could be the only blockchain ppl use eventually. @dana-edwards is still around, i think they just don't want to talk more about it until the testnet.
Interesting distinction. It's almost like the traditional distinction in formal logic, deductive vs inductive logic. One gives definite results but can only give you what it already knows. The other relies on observed patterns but may be biased if the patterns are not representative of the whole.
check out tau.net :)
At the end I think logical AI still pretty look more interesting than statistical AI
This post has been manually curated by @steemflow from Indiaunited community. Join us on our Discord Server.
Do you know that you can earn a passive income by delegating to @indiaunited. We share more than 100 % of the curation rewards with the delegators in the form of IUC tokens. HP delegators and IUC token holders also get upto 20% additional vote weight.
Here are some handy links for delegations: 100HP, 250HP, 500HP, 1000HP.
100% of the rewards from this comment goes to the curator for their manual curation efforts. Please encourage the curator @steemflow by upvoting this comment and support the community by voting the posts made by @indiaunited.
That's why my main concern with Statistical AI is that It can be molded into having "biased" outputs assigned to It.
I remember when I was first taught about the concept of AI. The teacher told me "When you're coding you have to write the functions and methods to get the outputs you want from the inputs you enter. When you're working with an AI, you enter the inputs and the outputs you want to get, and the AI codes the functions".