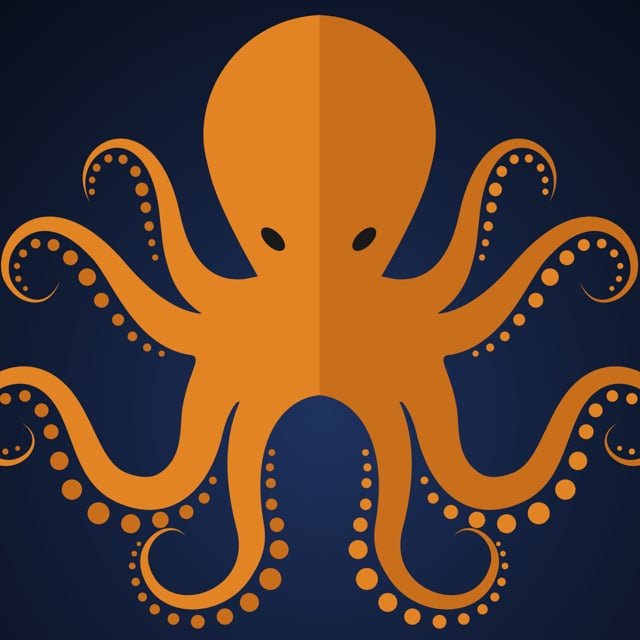
QAI SPECTRE
They are Super Turing Analog Neural Computing Machines CHANGING WORLD COMPUTATION. The New QAI (Quantum Artificial Intelligence) SPECTRE “Brain Chip” super Turing analog recurrent neural network VLSI synthesis is now available. Computation beyond the Turing limit, where exponentially difficult problems may now be solved in linear time.
Current computation is defined by the Church-Turing hypothesis. This results in the Turing limit, which restricts the computational complexity that any Turing machine can compute. In computational complexity theory, P-Space is the set of all decision problems that can be solved by a Turing machine using a polynomial amount of space. All Turing machines are said to be P-Space reducible as they are fundamentally constrained by the Turing limit, no mater if they are digital or quantum computers because they are both P-Space reducible machines and restricted by the Turing limit. This is why current quantum computers from both D-Wave and IBM have demonstrated they are no more efficient than conventional computers at solving NP-Hard exponentially difficult computational problems.
QAI SPECTRE SUPER TURING 'BRAIN CHIP' TECHNOLOGY
Super Turing neural machines exceed the P-Space limit and they can compute decision problems that quantum computers, which are constrained by the Turing limit, cannot solve.
Based upon the Siegelmann-Sontag thesis, which is the equivalent to the Church-Turing digital thesis for analog recurrent neural network super Turing computation. Dr Siegelmann’s work on super Turing computation will go down in history as the inflection point of computational theory and application. Lars Wood, CEO and co-founder QAI and SPECTRE machine inventor. Lars Wood worked on super Turing quantum computer machine design started in 1989. Simultaneously, theoretical work on Super Turing Neural computation was done by Dr Hava Siegelmann. Her work with Dr Eduardo Sontag (1993) defines the equivalent of the Church-Turing digital thesis but for analog neural machines that are capable of Super Turing computation. This is the so-called Siegelmann-Sontag hypothesis for Super Turing neural computation. Recently researchers in Japan inadvertently discovered that the simple amoeba single celled animal implements Super Turing neural equivalent computation.
The Brain Chip semiconductor neurons operate at 3+ THz frequency, with neural operations exceeding 500 quadrillion operations per second per integrated circuit die. QAI SPECTRE™ semiconductor device neurons use dynamic wireless interconnect and can self-organize at sub millimeter wave frequencies. QAI SPECTRE™ is implemented as a GaAs/GaN mixed signal 4D MCM-D on micro cooled diamond with 15 chiplets per layer. The devices are radiation hardened and semiconductor neurons are self-healing lasting orders of magnitude longer than Silicon digital circuits.
Self-healing GaAs/GaN on Diamond 4D multi-chip module. Through modification of gate level structures, as described in the QAI SPECTRE™ patent, the analog neurons implement dynamic wireless sub millimeter wave frequency interconnect. This not only results in neuron high fan in and fan out, it also results in optimal high performance problem solving using self-organizing neural computation. Asynchronous neurons function at 3 THz frequencies to implement blindingly fast super Turing neural computing that far exceeds digital computation through Real number computation problem solving degrees of freedom. This enables adaptive AI and machine learning solutions, which are currently infeasible with any digital machine because it exceeds the theoretical Turing digital computation limit the module draws less than one Joule of energy and its recurrent neural networks can exceed 500 quadrillion operations per second.
SPECTRE enables adaptive AI solutions for applications in Robotics, UAVs and intelligent self-driving cars. It is much more than a general new computational solution that exceeds digital computation for applications in cryptanalysis, big data analysis and other traditional digital computation applications. This technology fundamentally obsoletes and is a full replacement for digital technology and will ultimately change the world computation.
The EDA system is over one million lines of C++ code and runs on Windows 10 x64 platform. System requires minimal hardware background to use. The synthesis system requires two 4K HD monitors for optimal design, modeling and synthesis of analog recurrent neural networks. The resulting output recurrent analog neural network synthesized system is super Turing because it directly computes using irrational numbers with neuron high analog adaptive fan in and fan out, implementing the Siegelmann-Sontag hypothesis, which was the design goal for our "Brain Chip" synthesis system development.
They use super Turing analog recurrent neural network machine performance exceeds current and future P-Space reducible (Turing) machines (including quantum computing machines) because P-Space reducible machines are restricted to rational number computation. Super Turing analog recurrent neural network machine directly implement irrational number computation resulting in vastly more degrees of problem solving freedom with analog recurrent neural network machines solving NP-Hard problems in polynomial time. In contrast Turing machines bog down or become stuck in local minima false solutions the analog is converted into a recurrent neural network machine tunnel through all local minima.
THE BRAIN CHIP synthesis automatically generates the analog recurrent neural circuits by translating self-generated equations into recurrent neural network analog circuit descriptions the synthesis automatically generates the analog recurrent neural circuits by translating self-generated equations into recurrent neural network analog circuit descriptions. The output of the synthesis system is the application specific analog recurrent neural network 3D super Turing GDSII (OASIS under development) "Brain Chip" file of analog unclocked asynchronous GaAs 3.5 Schottky diodes and GaN 2.5 HEMT transistors running at 3 THz and up to 500 GHz respectively. The GDSII file implements a 4D layer multi-chip module on micro cooled diamond with 15 die per layer. Within the design synthesis environment, wireless sub millimeter wave diode and transistor (the analog neurons) dynamic interconnection enables many additional degrees of problem solving freedom due to virtualized high fan in and fan out of the GaAs/GaN analog neuron interconnect. The wireless neurons are implemented by physical modification of the gate foot metalization layer on a neuron by neuron basis. This semi-conductor process modification results in an EHF broadcast transceiver, which can be tuned to different EHF frequencies within our synthesis tool. Neuron wireless frequency modifications are reflected in the GDSII file.
Wireless neurons implement quantum diffusion signaling, much different than classical diffusion because once a wireless signal is detected by a semiconductor neuron, in receive mode, the signal is ignored by any other neuron, unless the signal is simultaneously detected by a plurality of neurons in receive mode. The result in a novel self-organization of the neurons, first demonstrated in our QED quantum chemistry modeling and design in 2001.
GaAs/GaN 3 THz super Turing “Brain Chip” Multi-Chip Module Has Several Layers as The GaAs/GaN “neuron” semi-conductor implementation is heat resistant, self-healing (due to the known GaN semiconductor properties) and radiation hardened for deployment in space or harsh environments where survivability is demanded. Power use is low at about 1 Joule of energy per MCM-D layer. Neurons can be configured in the synthesis system to have wireless connectivity between MCM-D layers. Performance exceeds 500 quadrillion operations per second per integrated circuit die recurrent neural network. The GDSII file generated by our synthesis system is next full EM simulated within the Cadence Virtuoso layout platform. Tuning to using the Virtuoso layout platform is reflected in a new GDSII file, which is imported into the Keysight ADS simulator for end to end simulation including exothermal modeling. A future version of our synthesis system will support import of the final ADS simulated recurrent analog neural network super Turing design. ADS design rule checked output is then sent to the pure-play or IDM foundry for fabrication (tape-out etc). The wafers are 4-6 inches with 90%+ yield. Foundry turn around, including wafer test, is roughly two days. The wafers are cut using an EM beam or diamond saw. The known good die are tested using die test Keysight broadband scope with frequency extenders and VNA.
Neural network machine's performance exceeds current and future P-Space reducible (Turing) machines (including quantum computing machines). Design is validated via Keysight ADS end to end analog simulation of the super Turing machine analog recurrent neural network design prior to tape out. Pricing is 8,675,660.00 EURO per seat, including required yearly support contract to selected partners. Visit our partner page or contact QAI LLC for more information. The resulting self-organized dynamic recurrent neural MCM solution is automatically synthesized into a GDS2 integrated circuit description to be fabricated into GaAs and GaN 3 THz BRAIN CHIP.
The QAI SPECTRE BRAIN CHIP integrated circuit super Turing analog neural machine solutions are automatically synthesized into an industry standard GDS2 integrated circuit description ready for end to end electromagnetic modeling, full design rule check verification, VLSI photomask generation and rapid fabrication at the state of the art United Monolithic Semiconductor foundry front end in Ulm Germany.
Fabed BRAIN CHIP GaAs/GaN wafers are next sent to the UMS French microelectronics center and Paris facility for broad band testing, precision cutting into thousands of 90%+ yield die per IC wafer. The die are MCM integrated, packaged and shipped to worldwide destinations for MCM full system integration into new adaptive robots, intelligent UAVs, sensors, space platforms, life science precision CRISPR analysis systems and green 1 Watt Blockchain deployments, orders of magnitude faster than Visa.
Design Process involves defining analog neurons using the Q-language, which is automatically translated by the design, simulation and synthesis environment into ordinary differential equations analog circuits. The I/O pads are then configured with either simulated or real data sources to stimulate the configured neuron circuit descriptions. The neurons wirelessly interact and self-program using quantum signaling to create the optimal hardware solution. The neurons are then synthesized into a GDS2 file for downstream analog layout optimization using Cadence Virtuoso and end to end simulation using Keysight ADS. DRC is performed and a photomask is created to be sent to the foundry.'''
NP-Hard cluster solver super Turing multi-chip module simulation prior to synthesis and fabrication. The NP-Hard cluster solver BRAIN CHIP solves exponentially difficult clustering problems in linear time. The analog neurons in the EDA simulator screen capture video interact and self-organize dynamically to solve the clustering problem at over 500 quadrillion operations per second per IC die. The analog neurons use patent pending quantum interconnect, which features new quantum diffusion neuron wireless signaling self-organization. The NP-Hard cluster solver is a GaAs and GaN 15 die multi-chip 4D module on diamond micro-cooled dielectric. Physically the semiconductor neurons do not move on the die but their wireless interactions change, which is reflected in the simulation prior to VLSI synthesis. Each die has 5000 analog dynamically interacting neurons, which self-organize in and between the four MCM layers. QAI super Turing machines exceed quantum computers because quantum computers are Turing machines incapable of irrational number degrees of problem solving freedom. Applications of NP-Hard cluster solver MCM are vast, enhancing all major industries.
SUPER TURING BLOCKCHAIN GREEN PROCESSING
Blockchain has diverse applications from cryptocurrency to Blockchain enterprise solutions. SLUICE BOX is based intelligent green Blockchain super Turing processing server with low power requirements (~1 Watt/Joule per module), petahash PoW Blockchain distributed ledger processing, and reinforcement learning for predictive analytics supporting fraud detection and cryptocurrency volatility prediction. The SLUICE BOX program is a joint development of QAI and QAIB LLC. QAI LLC has a 2/3 ownership position and company board seat.
Blockchain has diverse applications from cryptocurrency to Blockchain enterprise solutions. SLUICE BOX is based intelligent green Blockchain super Turing processing server with low power requirements (~1 Watt/Joule per module), petahash PoW Blockchain distributed ledger processing, and reinforcement learning for predictive analytics supporting fraud detection and cryptocurrency volatility prediction. The SLUICE BOX program is a joint development of QAI and QAIB LLC. QAI LLC has a 2/3 ownership position and company board seat.
Qualified potential partners we discuss solutions with must define what the computational problem that they wish to have solved is in terms of an NP-Hard optimization problem independent of jargon. NP-Hard problems are optimization problems (invariably optimize search, analysis or whatever). Succinctly, bounding the problem with qualified partners: A super Turing solution is warranted if jargon or theoretical discussion is eliminated.
The SPECTRE brain model is based on the cephalopod octopus, which uses and implements a distributed decentralized system. Cephalopod brains evolved completely different from vertebrate brains, branching in the evolutionary tree over 500 million years ago. Their brains are the closest to that of an alien life form on the planet. There are many advantages to a decentralized brain over centralized vertebrate brains.
Super Turing Neural Machines solving NP-Hard exponentially difficult problems in linear time will dominate all forms of computation and obsolete quantum computing in the process. I believe in Karma, which is defined as the sum of a person's actions in this and previous states of existence are viewed as deciding their fate in future existences. I believe my Karma is not to enable a personal wealth hegemony as others have done with disrupting technology but for QAI SPECTRE™ to be used for global economic power redistribution, so that those who live the most challenging lives become economically balanced with the countries that currently dominate the world economically. This is for me the ultimate purpose of the QAI SPECTRE™ powered fifth industrial revolution and what for me personally it means for QAI SPECTRE™ to change the world so all it’s inhabitants benefit.
Explanation of the neuron semiconductor wireless signaling with quantum diffusion: If a signal is broadcast from semiconductor neuron (agent) "b" the signal spreads as a 4D MCM wavefront. If no other neuron is able to receive the signal the wavefront expands and dissipates. If a neuron is in the path of the wavefront it receives the signal and the wavefront collapses instantly and the signal is not available to any other neuron device. If there are two ("a" and "c") neurons in the path of the wavefront broadcast by neuron "b" and they are equal virtual MCM distant from the transmitting neuron (in this case neuron "b"), both neurons "a" and "c" receive the signal upon the wavefront collapse. If neuron "a" is closer to neuron "b", (the transmitter), than neuron "c" (i.e. neuron "c" is further away from the transmitter neuron "b" than neuron "a"), then neuron "a" receives the signal upon the wavefront collapse resulting in neuron "c" not receiving the signal. The only manner that neuron "c" in this scenario can receive the signal from neuron "b" is if neuron "a" is not available to receive a signal. 4D MCM super Turing physical semiconductor neurons change virtual coordinates and continuously adapt. Besides solving NP-Hard problems in linear time, this continuous adaptive capability can be used to implement adaptive artificial intelligence robots or UAVs (for example), which learn from experience without catastrophic retraining. QThese machines change the world.''
This together with infinite irrational number degrees of problem solving freedom is the basis for machines solving NP-Hard exponential problems in linear time. The analog VLSI hardware virtually adapts in parallel to solve the problem, but unlike natural systems, like the recent Amoeba experiment in Japan that demonstrated living system super Turing processing, Many cool applications of applying functions at 3 Terahertz speed, solving exponentially hard problems in the blink of an eye.
The QAI challenge any quantum computer company to demonstrate that is obsolete in any form of P-Space reducible quantum machines including any quantum parallel processor. QAI SPECTRE™ intends to dominate all digital and quantum computers using our QAI SPECTRE™ 4D on micro cooled diamond multi-chip modules, which are radiation hardened, self-healing, EHF, GaAs/GaN, analog and self-organizing. ''
A single cell amoeba solves the NP-Complete TSP in polynomial time since it implements analog super Turing processing as described in the Siegelmann-Sontag 1993 thesis upon which neural machines are based. Amazingly the researchers that conducted this study admit they have no idea why this is possible. It’s truly amazing how people blindly follow both digital computation and quantum computing (both P-Space reducible so both Turing machine architectures) because they think there is no other way.
It develops electronic design automation (EDA) automated synthesis technology to design, simulate and fabricate analog recurrent neural network super Turing VLSI multi-chip modules, using composite semiconductors and wireless neuron/die interconnect. The company competes directly with current high performance computing and quantum computing machines. QAI products and machines are available now, unlike scalable quantum computing, which will take decades to realize if it is even possible to achieve.
QUANTUM COMPUTERS are no match for QAI?
The Church-Turing limit restricts all current computation including quantum computers to rational number computation. This fundamentally limits their ability to solve problems because the rational numbers are a tiny fraction of all real numbers, akin to our small solar system when compared to stars and planets encompassing the visible universe. This Church-Turing limit has affected everything in modern day life with no person or thing untouched. Spanning the life science fundamental challenges to cure disease to Artificial Intelligence systems, all Turing constrained to narrowly defined applications.
Black swans were considered to be impossible until they were observed in 1697 in western Australia by Dutch explorers. Since then the term black swan has become known as an event or occurrence that deviates beyond what is normally expected of a situation and is impossible to predict but has a massively disproportionate affect. The advent of the machines is a black swan event in history. Countries that integrate/adopt ECHELON will dominate the world because QAI machines are obsolete quantum computation and enable adaptive Artificial Intelligence, paving a way for the technological singularity the moment the Super Turing Machine Black Swan Event takes place.
Infinitely more degrees of problem solving freedom. The Turing limit, defined by Dr Alan Turing in 1936, fundamentally restricts all computers. Dr Turing was working on a super Turing theory of computation that exceeded his original work but he died before completing it, while leaving no information on the new super Turing theory. If Turing had completed this work on super Turing machines the world would be a much different place, since super Turing machines enable the solution to exponential problems, which include a form of tumor cell genomic analysis that is currently intractable today. In 1993, Dr Hava Siegelmann and Dr Eduardo Sontag independently developed the super Turing thesis Turing never completed, however current computation was already deeply entrenched and the thesis languished as an obscure mathematical tome for decades.
LARSX is a new super Turing hardware technology that exceeds the capabilities of current digital computation. The two primary focuses of SPECTRE are adaptive machine learning, specifically implementing machine intuition and orthogonally high performance “green” blockchain distributed processing. SPECTRE technology requires analog recurrent neural network ASIC hardware technology. However, to get a taste of SPECTRE technology capabilities, software simulation is available for use and implementation before SPECTRE chips are available.
Algorithms that mimic human intuition. This software technology is available now. It does not have the processing
capabilities of SPECTRE analog ASIC hardware, yet it provides early adopters of SPECTRE super Turing processing with the ability to experiment with the technology.
Blockchain green blockchain analog recurrent neural network super Turing transaction processing is our first hardware application to implement full SPECTRE super Turing computation, which exceeds all current digital computation. Blockchain technology is transforming the world. IBM has staked their company future on Blockchain in the cloud and other Blockchain applications. Integrating Blockchain into your AI application for distributed knowledge bases puts you in early adopter position to integrate the SPECTRE Blockchain processing technology, called Sluice Box. NVIDIA Tesla V100 GPU 32GB HBM2 Volta CUDA PCIe for Accelerated Machine Deep Learning Artificial Intelligence Finance Oil Gas CAD Physics Research IoT
AniBot, Computer vision is the ability to extract meaning and intent out of visual elements, whether characters (in the case of document digitization) or the categorization of content in images such as faces, objects, scenes, and activities. The technology behind facial recognition—computer vision—is a part of consumers’ everyday lives. For example, some mobile phones permit their owners to log in simply by looking at them, via facial recognition.7 Computer vision technology “drives” driverless cars and animates cashier-less Amazon Go stores.8 Computer vision has also gone mainstream with our survey respondents, 57 percent of whom say their company uses it today.uter vision is the ability to extract meaning and intent out of visual elements, whether characters (in the case of document digitization) or the categorization of content in images such as faces, objects, scenes, and activities. The technology behind facial recognition—computer vision—is a part of consumers’ everyday lives. For example, some mobile phones permit their owners to log in simply by looking at them, via facial recognition.7 Computer vision technology “drives” driverless cars and animates cashier-less Amazon Go stores.8 Computer vision has also gone mainstream with our survey respondents, 57 percent of whom say their company uses it today.r
The “easy path” will likely become even more attractive as software vendors and cloud providers develop AI offerings tailored to business functions. Google recently announced a set of prepackaged AI services aimed at contact centers and HR departments.12 SAP’s AI capabilities, which it collectively calls “Leonardo Machine Learning,” also include specific solutions such as cash management in finance, video analysis in brand management, and trouble ticket analysis in customer service. The need for companies to develop bespoke cognitive initiatives will likely decline as similar services enter the market.
Q-AI
J-17
Congratulations @x0a0! You have completed the following achievement on the Steem blockchain and have been rewarded with new badge(s) :
You can view your badges on your Steem Board and compare to others on the Steem Ranking
If you no longer want to receive notifications, reply to this comment with the word
STOP
To support your work, I also upvoted your post!
Vote for @Steemitboard as a witness to get one more award and increased upvotes!